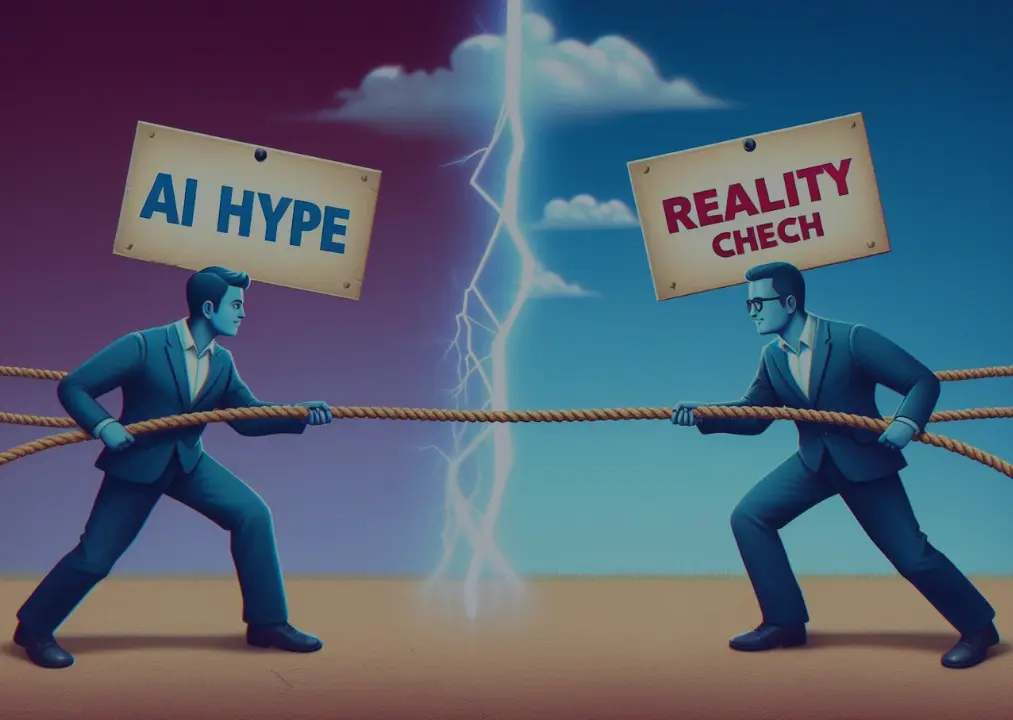
AI broke the internet. Tools like ChatGPT stunned the world overnight. It could write poetry, solve riddles, and answer questions with shocking speed. Everyone thought the future had arrived.
But under the surface, a hard truth is emerging. The idea was simple: keep feeding AI more data and computing power, and it would just keep getting smarter. But that idea might be breaking down.
👉 Prefer to watch? Check out the full video version of this article on our YouTube channel: 🎥 AI Reality Check – Watch Now
The Dream of Limitless Growth
At first, it looked unstoppable. Big tech companies raced to build faster, smarter, and more powerful AI. Investors poured billions into the industry, expecting huge returns. Everyone wanted a piece of the next big breakthrough.
And for a while, they got it. AI systems improved quickly, doing things that once seemed impossible. People imagined machines that would keep learning forever—machines that would transform the world.
But Then—The Wall
Now, that progress is hitting roadblocks. Training large AI models is incredibly expensive. We’re talking millions of dollars just to teach one system how to respond better. And even after all that, the improvements are getting smaller.
That magical leap from GPT-3 to GPT-4? Impressive, yes—but nowhere near as big as the leap from GPT-2 to GPT-3. It’s like climbing a mountain where the higher you go, the harder it gets.
A History of Hope and Hype
The dream of AI isn’t new. It goes all the way back to the 1950s when Alan Turing asked: Can machines think? His famous Turing Test became the first serious attempt to measure machine intelligence.
But real progress was slow—too slow for many. That’s why AI went through what scientists now call “AI winters,” where the hype died down and funding dried up. Now, some experts wonder if we’re entering another winter, not because AI has failed, but because it’s finally facing reality.
What’s Holding AI Back?
One word: limits. We’re hitting limits in three major areas:
- Data – AI needs vast amounts of clean, labeled information. But we’re running out of high-quality, fresh data to train on.
- Compute Power – Training large models requires powerful hardware, and the cost is exploding.
- Understanding – No one fully knows how large language models learn what they learn. They’re still black boxes, no matter how impressive they seem.
Some researchers warn that this might not be a temporary pause. It could be a sign that we need to rethink how we build and measure AI.
A Shift in the Industry
This slowdown isn’t the end—it’s a turning point. AI isn’t going away. But the wild pace of discovery might be. Startups and investors may have to adjust expectations.
The next wave of innovation might not come from “bigger is better.” Instead, it could come from smarter design, clearer goals, and more responsible development.
Final Thoughts
We’ve seen this story before: fast progress, big promises, and then a moment of truth. That moment is now. AI has amazed the world, but it’s not magic.
It’s time for an honest AI reality check.
🎥 Subscribe to our YouTube channel: Growing Technology for more insights into this tech revolution.
Found this helpful? Share the wisdom!